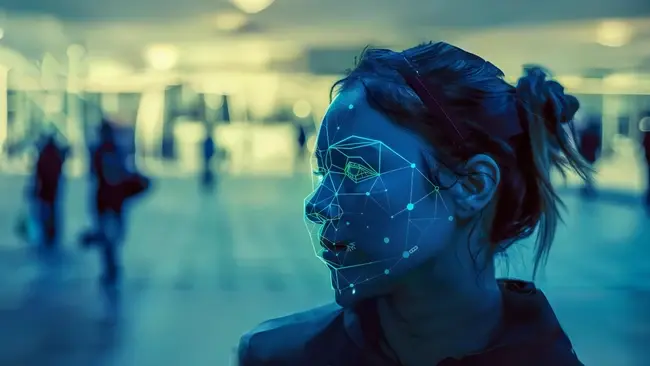
Artificial intelligence (AI) is increasingly impacting critical business areas, including recruitment, healthcare, and sales. So it’s not a surprise that one question continues to linger: are AI algorithms biased?
The short answer is yes! AI is biased because the people who train it with data are biased. These biases can be implicit, societal, or caused by underrepresentation in data, which can be damaging to organizations. I
t doesn't matter how powerful the AI is or how big the company behind the AI is either. Google, one of the leaders in AI development, was recently called out after its large language model (LLM), Gemini, appeared to portray bias for particular ethnicities when it generated images.
OpenAI's ChatGPT has also been called a "woke AI" by high-profile figures including Elon Musk due to it supposedly having a bias towards certain values and political ideologies.
If customers get the idea that a company’s algorithm is prejudiced, it can turn them away from its product. That means lost revenue and a damaged reputation. So, how can you prevent AI bias?
Unfortunately, the most effective way to get unbiased AI systems is to have unbiased humans - which is pretty near impossible. However, there are several strategies you can follow - keep reading to discover them.
What is AI Bias?
AI bias, also sometimes called machine learning bias or algorithm bias, refers to situations where AI systems produce results that are prejudiced or unfair due to assumptions during the machine learning (ML) process.
AI systems are trained on massive datasets. If this data contains inherent biases, like reflecting historical prejudices or social inequalities, the AI system will learn those biases and incorporate them into its decision-making.
For instance, an AI system used for hiring might favour resumes that use traditionally masculine terms like "executed" or "captured" because these words were more common in past successful applications, even though those terms may not be relevant to the job itself.
The way AI algorithms are designed can also introduce bias. For example, an algorithm that relies heavily on past data to predict future outcomes may amplify existing biases. Imagine a system used to predict loan approvals.
If historically, loans were denied to people in certain neighbourhoods, the algorithm might continue to deny loans to people from those areas, even if their creditworthiness is good.
AI system. The results of AI bias can range from annoying to harmful. For example, a biased language translation system might portray one culture in a negative light. In a more serious case, a biased hiring algorithm could unfairly screen out qualified candidates.
That's why the field has become such important area of research, as experts try to develop methods to mitigate bias in AI systems.
Types of AI Bias
Let’s take a look at three common types of AI bias. They are:
1. Prejudice bias
This occurs when the training data contains existing prejudices, societal assumptions, and stereotypes. As a result, these biases are rooted in the learning model.
For example, Amazon discontinued its hiring algorithm when it realized it systematically discriminated against women applying for technical jobs, such as software engineer positions.
But this wasn’t a surprise. Amazon's existing pool of software engineers was overwhelmingly male at the time, and the program was fed resume data from those engineers and the people who hired them.
2. Sample selection bias
Sample selection bias is a result of the training data not being a representation of the population under study. Imagine an AI system trained to detect skin cancer. If it’s trained mostly on images of white skin, it’ll underperform when applied in the real world. This could lead to poorer healthcare outcomes for groups that weren’t represented in the data set.
3. Measurement bias
This bias occurs due to an error in the data collection or measurement process.
For example, in 2019, researchers discovered that an algorithm used in US hospitals to predict additional healthcare needs heavily favored white people over black people. This happened because the algorithm was trained to predict healthcare needs based on patients’ past healthcare expenditures.
White patients with similar diseases spent more than their black counterparts, so the algorithm heavily favored them.
Now that you know what AI bias is and the common types, let’s discuss how to mitigate them.
10 Ways to Prevent AI Bias
In a perfect world, you can totally prevent AI bias. You can rid your training dataset of conscious and unconscious suppositions on gender, race, or other perspectives, you can develop an unbiased AI system.
But it all comes down to one simple fact: an AI system is as good as the quality of data it receives. Humans are the ones who input data into these systems, and unfortunately, we’re biased.
On the bright side, we can reduce this by implementing some of the tips above and paying close attention to what our AI models are saying.
Here are ten key steps organizations developing AI must take to reduce AI bias across their systems.
Take feedback on board
Even with the best of intentions, some bias may slip through the system. This is where being transparent can be beneficial, as it shows users you’re aware of any systematic preferences and are actively seeking to prevent or overcome them.
Those same people are also part of the resolution. Use the data you have to hand to factor in their backgrounds and perspectives when building your model to ensure it’s trained appropriately. Gather feedback from end-users too, as they’re most likely to pick up on any unfairness or discrimination. Issuing a simple survey is enough to gauge their perception. By reviewing their experience with your AI, you can identify issues and adapt your model to meet their needs.
Conduct tests in a real-life environment
You may think your AI is unbiased in theory, but how does it stand up in practice? The only way to find out is to test the algorithm in a real-world setting.For example, let’s say you provide video conferencing software for large conference room setups. The system uses AI-powered facial recognition to frame meeting participants depending on who is speaking.
Before launching the product and even after taking it to market, put it to the test. Gather your diverse team and ensure there are no discrepancies in how the software identifies different people. Regularly monitor these capabilities by reviewing results in real time, and ensure they align with client experiences and feedback, too. If you solely test your AI’s accuracy in one setting, it may skew the results.
Consider human-in-the-loop systems
It is important to recognize the limitations of your systems. Your data, models, and technical solutions are unlikely to eliminate the risks of unwanted bias – especially with biased data. That’s why you need to include a human in the loop! The purpose of human-in-the-loop is to achieve what neither a computer nor a human being can achieve for themselves. This strategy is typically deployed when training an algorithm, such as a computer vision model.
Data scientists and human annotators can offer feedback that enables the models to have a better understanding of what is being shown. Imagine you’re using machine learning as part of your IT risk management process, and you specifically want to train a spam filter to look for phishing attempts. You may find the system assumes misspellings means something is spam, when in fact it can just be human error. Providing constant feedback can help the system learn and enhance its performance after each run.
Set standards
Preventing AI bias requires training your AI models with quality and representative data. To accomplish this, you must develop a policy and framework for collecting, sampling, and pre-processing training data. Additionally, it's worth considering incorporating an AI post generator into your workflow. These tools can assist in generating diverse datasets that reflect a broader spectrum of societal demographics, thus reducing the risk of bias in training data.
This is because ensuring unbiased AI demands meticulous data handling. So, you need tools like Apache Hive to effectively process your data. For example, Apache Hive uses a structured query language, aiding in organized data storage. This is pivotal in implementing your strategy to prevent bias. Additionally, you may engage internal and external teams to identify issues like racial or gender biases in the data— dealing with them before they become a source of bias in the model.
Increase transparency
The lack of clarity with AI processes remains an issue. For instance, deep learning algorithms use neural networks designed like the human brain to make decisions. To understand where bias comes from, we need to know how neural networks arrive at their decisions.
That’s why the move toward explainable AI is so important. It aims to reveal how data is being trained and how we’re using different algorithms. Solutions like SQL spark encourage transparency in data analysis due to its declarative nature. The clear syntax makes it easier to document and audit queries used to prevent AI bias, fostering collaboration and trust. Of course, making AI explainable may not totally eradicate AI bias but it can certainly help with spotting the root causes.
Review the AI training data
It may seem obvious, but it is vital to understand your training data. Analyze your training dataset to determine if it is representative and large enough to mitigate common biases, like sample selection bias. AI training data review also helps developers understand how data has evolved. As a result, they can create more ethical, fair, and accountable AI systems. This is one area where a delta lake proves its worth.
A delta lake is a data storage layer that provides reliability, scalability, and performance optimizations for big data processing. It enables data versioning, allowing you to track changes. This way, you can ensure biases aren’t unintentionally embedded in your AI models. Imagine you have a loan approval model and notice it is suddenly denying loans to applications from a certain location. Looking at recent changes, you notice a new upload of training data from some of your banks. However, it only contains the rejections, unintentionally biasing your model. Tracking changes allows you to highlight the origin of the problem and resolve it.
Consider the context
While there are some situations where AI can actually help correct bias, others are more prone to bias. For example, in many legal and financial settings, there’s a clear history of biased systems and misrepresentative data.
So consider the context of your AI system.
- How is it being used?
- Is there a low or high margin for bias here?
- Was the system trained using skewed data?
- Can you access a wider data set to avoid this?
Keep in mind the definition of “bias” can also vary – that is to say, you need to explicitly tell the AI system how you define and measure fairness in different scenarios.
Design AI models with inclusion in mind
With a diverse team, you will be naturally inclined to design your algorithm to be devoid of bias. Collaborate with social scientists and ethicists to guide you in creating models that don’t inherit bias present in human judgment. In addition, set quantifiable goals for the AI models to have the same level of performance across intended use cases—for example, race or age groups.
Suppose you’re developing an AI model for a particular New Zealand domain. In that case, set benchmarks for performance across various demographics within that domain. Establish metrics that assess the model’s accuracy, fairness, and effectiveness across each statistical group.
Maintain a diverse team
The output of your algorithm is as good as its input. Preventing AI bias starts with creating a diverse team (in terms of demographics and skillset) to train the machine learning systems. A diverse AI team will offer different perspectives and easily identify and flag any bias in the development process. During virtual brainstorming sessions or team meetings, they can help to interrogate wider organizational processes that could be causing bias and affecting how your technology is developed.
Embrace the likelihood of AI bias
To prevent AI bias, the first thing you need to do is accept that there is bias in your algorithm. After all, the system was trained by humans, who are biased by default. You may be tempted to find a quick solution by removing protected classes such as race or gender from the data and deleting the labels that make the algorithm biased. However, this approach will fail because the model can build up an understanding of these protected classes from other labels, such as postal codes (proxy discrimination).
Also, removing these labels can impact the understanding of the model, and the accuracy of your results may become worse. Ideally, you should conduct a thorough analysis of the algorithm's training data and identify potential sources of bias. Then, you can implement strategies to mitigate and minimize bias in your algorithm. One such strategy includes improving diversity, which takes us to the next point.